Keynotes
Keynote 1 – Ali Sayed, EPFL
Social Machine Learning
A wide range of learning algorithms are available in the literature, including sophisticated structures that are based on feedforward, recurrent, or convolutional neural networks. The performance of these architectures matches or exceeds human performance in many important applications. However, they are susceptible to adversarial attacks that can drive them to erroneous decisions under minimal perturbations. They are also often trained with data that arise from homogeneous statistical distributions. And, once trained, the internal structure of these systems remains fixed and are expected to deliver reliable decisions thereafter. For all practical purposes, learning is turned off following training. Contrast these situations with learning by humans: they learn from different types of data and even minimal clues are sufficient in many instances. Humans are also more difficult to fool by small perturbations, and they continue to learn and accumulate experiences over time.
Motivated by these considerations, we will discuss one architecture for learning that exploits important characteristics of social interactions. We refer to the new framework as Social Machine Learning, and it consists of two main connected blocks. One block represents the memory component of the learning machine since it will learn the underlying clues, store them, and regularly update them. This ability adds a new level of richness to the learning process and is different from traditional boosting techniques because the processing is fully decentralized.
A second block represents the processing component of the social learning machine, and it consists of a graph structure linking the various clue models. This block performs classification by exploiting repeated social interactions among agents connected by a graph topology. The agents observe heterogeneous data arising from different statistical sources. This ability is different from neural network structures where information flows in a particular direction rather than arbitrarily over the graph edges, and where the feature data feeding into the graph is now highly heterogeneous. Moreover, the interactions among the agents on the graph take advantage of the “wisdom of the crowd” paradigm, which should lead to more robust learning. This is because it is more difficult to deceive a group of agents than an individual agent, especially when different parts of the group are observing different clues, not all of which can be perturbed similarly.
Analyses based on statistical learning theory indicate that, under reasonable conditions, the social machine learning structure can learn with high confidence. Moreover, the proposed architecture handles heterogeneity in data more gracefully, is able to learn with performance guarantees, is more resilient to attacks by exploiting the power of the group, and enables continuous learning.
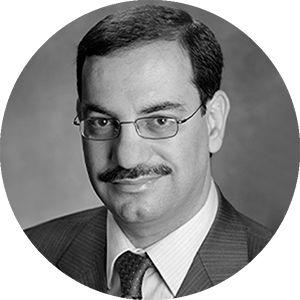
Ali Sayed
A. H. Sayed is Dean of Engineering at EPFL, Switzerland, where he also leads the Adaptive Systems Laboratory (https://asl.ep.ch/). He is a member of the US National Academy of Engineering (NAE) and The World Academy of Sciences (TWAS). He served as President of the IEEE Signal Processing Society in 2018 and 2019. His research areas cover adaptation and learning theories, data and network sciences, and statistical inference. His work has been recognized with several awards including the 2022 IEEE Fourier Award, the 2020 Norbert Wiener Society Award, the 2015 Education Award, and the 2012 Technical Achievement Award from the IEEE Signal Processing Society. He also received the 2014 Papoulis Award from the European Association for Signal Processing, the 2005 Terman Award from the American Society for Engineering Education, and several Best Paper Awards. He is a Fellow of IEEE, EURASIP, and the American Association for the Advancement of Science (AAAS).
Keynote 2 – Lee Swindlehurst, University of California Irvine, USA
Intelligent Reflection and Absorption for Sensing, Interference, and Energy Efficiency
Nearly passive metasurfaces have attracted great interest recently given their ability to tune the RF propagation environment and enhance the capabilities of wireless communication systems. Most work on such reconfigurable intelligent surfaces (RIS) has focused on designs that (almost) fully reflect all energy that impinges on the surface, in order to maximize performance metrics such as the network sum rate. More recently, focus has shifted to hybrid RIS architectures that sense or at least redirect some of the impinging RF energy in order for the RIS to (1) extract information for local processing (e.g., channel estimation), (2) refract some of it for transmission on the other side of the RIS, or (3) simply to absorb it. In this talk we will examine these alternative architectures and particularly focus on the advantages of partial absorption at the RIS for scenarios requiring interference mitigation.
Room: Aurora Hall
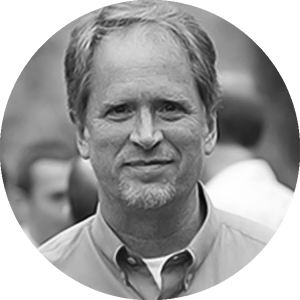
Lee Swindlehurst
Lee Swindlehurst received the B.S. (1985) and M.S. (1986) degrees in Electrical Engineering from Brigham Young University (BYU), and the PhD (1991) degree in Electrical Engineering from Stanford University. He was with the Department of Electrical and Computer Engineering at BYU from 1990-2007, where he served as Department Chair from 2003-06. During 1996-97, he held a joint appointment as a visiting scholar at Uppsala University and the Royal Institute of Technology in Sweden. From 2006-07, he was on leave working as Vice President of Research for ArrayComm LLC in San Jose, California. Since 2007 he has been a Professor in the Electrical Engineering and Computer Science Department at the University of California Irvine, where he served as Associate Dean for Research and Graduate Studies in the Samueli School of Engineering from 2013-16. During 2014-17 he was also a Hans Fischer Senior Fellow in the Institute for Advanced Studies at the Technical University of Munich. In 2016, he was elected as a Foreign Member of the Royal Swedish Academy of Engineering Sciences (IVA). His research focuses on array signal processing for radar, wireless communications, and biomedical applications, and he has over 300 publications in these areas. Dr. Swindlehurst is a Fellow of the IEEE and was the inaugural Editor-in-Chief of the IEEE Journal of Selected Topics in Signal Processing. He received the 2000 IEEE W. R. G. Baker Prize Paper Award, the 2006 IEEE Communications Society Stephen O. Rice Prize in the Field of Communication Theory, the 2006, 2010 and 2022 IEEE Signal Processing Society’s Best Paper Awards, the 2017 IEEE Signal Processing Society Donald G. Fink Overview Paper Award, and a Best Paper award at the 2020 IEEE International Conference on Communications.
Keynote 3 – Prof. Andreas Molisch
Propagation channels for 6G and why they matter for signal processing
With the ongoing deployment of 5G systems, the attention of the research community is turning to the next generation, 6G. One of the first steps in investigating new systems is the analysis of the propagation channels that they are operating in, and the requirements of 6G will create a lot of new scenarios that need to be investigated: from new frequency range (e.g., Terahertz), to new ways of deployment (e.g., distributed massive MIMO), to new mobility models (e.g., base stations mounted on drones). It is axiomatic that efficient signal processing needs to be tuned to, and exploit, the special properties of the propagation channels. This talk will thus survey the measurements and models in the new types of propagation channels and discuss their impact on various types of signal processing, from channel estimation algorithms to scalable MIMO decoding to computations with low-resolution ADCs. A discussion of some open topics will wrap up this talk.
Room: Aurora Hall
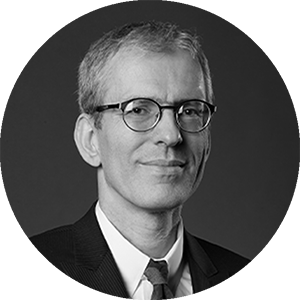
Andreas Molisch
Andreas F. Molisch received his PhD and habilitation from TU Vienna in 1994 and 1999, respectively. After 10 years in industry he joined the University of Southern California, where he is now the Solomon Golomb – Andrew and Erna Viterbi Chair Professor. His research interest is wireless communications, with emphasis on wireless propagation channels, multi-antenna systems, ultrawideband signaling and localization, novel modulation methods, machine learning, caching for wireless content distribution, and edge computing. He has published five books and more than 650 research papers, which have been cited more than 58,000 times (h-index >100); he has also authored numerous standards contributions and been granted 70 patents. He is a Fellow of the National Academy of Inventors, IEEE, AAAS, and IET, as well as Member of the Austrian Academy of Sciences and recipient of numerous awards.
Keynote 4 – Wen Tong CTO, Wireless Network, Huawei Technologies Co., Ltd.
Native-AI Enabled 6G Networks – Challenges and Design Aspects
AI and 6G are perfect storm that will revolutionize all the services and applications in 2030. With the success of the machine-learning technology and the potential for massive application based on the deep learning technology, we have the opportunity to re-architect the wireless networks to enable the native-AI rather than the add-on-AI. In this talk, we address three areas of the novel native-AI based 6G wireless designs: (1) AI based end-to-end link with a novel deep pre-coded transmitter and receiver for the real-world wireless fading channel, (2) the in-network learning and in-network inferencing, here, we present the methodology and the evaluation on the network and computing key performance Indicators (KPI) (3) the 6G data governance framework for raw-data-set collected from the billions of devices; that is, the deep learning model created by training and utilized for inferencing, we present a new principle as “ model-follows-data” and its relation with information bottleneck theory. Finally, we will address how to minimize the carbon emission associated with in-network computing for deep learning.
Room: Aurora Hall
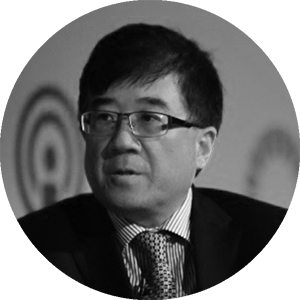
Dr. Wen Tong
Dr. Wen Tong is the CTO, Huawei Wireless. He is the head of Huawei wireless research. In 2011, Dr. Tong was appointed the Head of Communications Technologies Labs of Huawei, currently, he is the Huawei 5G chief scientist and led Huawei’s 10-year-long 5G wireless technologies research and development.
Prior to joining Huawei in 2009, Dr. Tong was the Nortel Fellow and head of the Network Technology Labs at Nortel. He joined the Wireless Technology Labs at Bell Northern Research in 1995 in Canada.
Dr. Tong is the industry recognized leader in invention of advanced wireless technologies, Dr. Tong was elected as a Huawei Fellow and an IEEE Fellow. He was the recipient of IEEE Communications Society Industry Innovation Award in 2014, and IEEE Communications Society Distinguished Industry Leader Award for “pioneering technical contributions and leadership in the mobile communications industry and innovation in 5G mobile communications technology” in 2018. He is also the recipient of R.A. Fessenden Medal. For the past three decades, he had pioneered fundamental technologies from 1G to 5G wireless with more than 530 awarded US patents.
Dr. Tong is a Fellow of Canadian Academy of Engineering, and he serves as Board of Director of Wi-Fi Alliance.
Keynote 5 – Gerhard P. Fettweis
On The 6G Radio Access Gearbox PHY Idea
When switching from odd to even-numbered generations, cellular standards have introduced a revolutionary change in the radio access network. The main reason is that odd-numbered generations break ground for a new communications paradigm, and even-numbered ones drive cost and energy down to democratize this for consumers. This requires the radical changes in radio access we have experienced. Looking forward, a breakthrough is required for 6G radio access, which also supports features such as “sensing as a service”. The good news is that we can exploit the vast differences in how data requirements are to be serviced over the area as well as over the time (24/7). This allows for a possible solution – the “Gearbox PHY”, with gears spanning from supporting extreme data rates down to a gear with extreme energy efficiency using analog impulse radio. The Gearbox PHY is to always serve the service needs in an energy optimal way.
Room: Aurora Hall
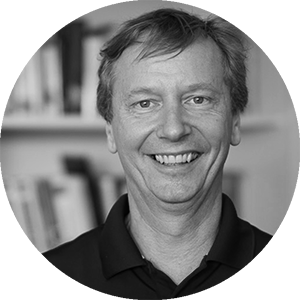
Gerhard P. Fettweis
Gerhard P. Fettweis is a Vodafone Chair Professor at TU Dresden since 1994 and the founding director of the Barkhausen Institute since 2018. He earned his Ph.D. under H. Meyr from RWTH Aachen in 1990. After being a postdoc at IBM Research, San Jose, CA, he moved to TCSI Inc., Berkeley, CA. He coordinates the 5G Lab Germany. In 2019 he was elected into the DFG Senate. His research focuses on wireless transmission and chip design for wireless/IoT platforms, with 20 companies from Asia/Europe/US sponsoring his research. He also serves on the board of National Instruments Corp, and advises other companies. Gerhard is a member of the German Academy of Sciences (Leopoldina), the German Academy of Engineering (acatech), and received multiple IEEE recognitions as well as the VDE ring of honor and the Semi Europe award. In Dresden, his team has spun out nineteen start-ups, and set up funded projects in volume of close to EUR 1/2 billion